Earth Observation
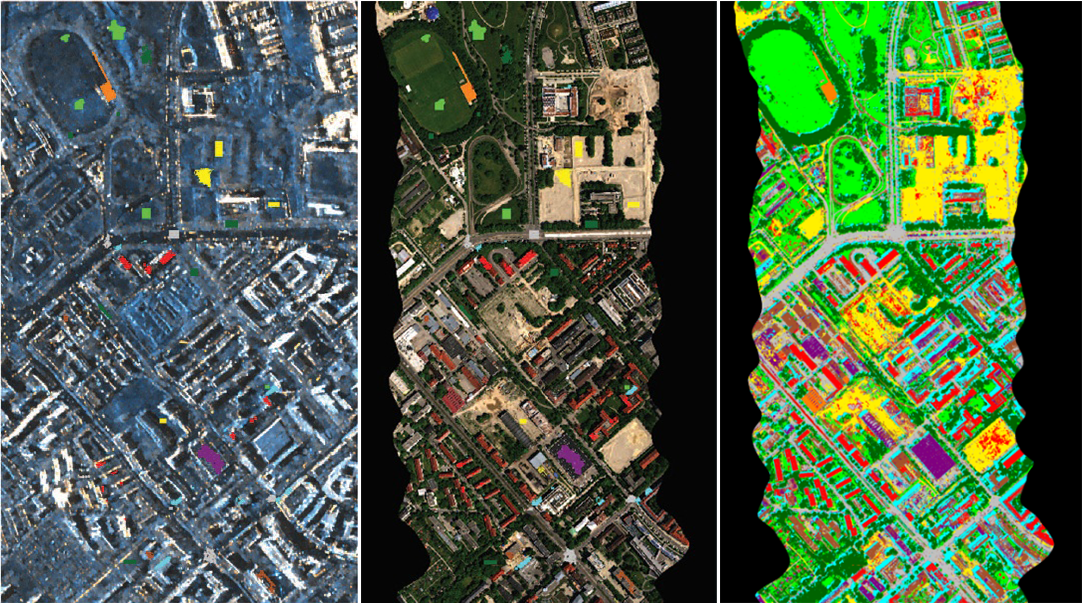
This use case focuses on the classification of global Local Climate Zones (LCZs). Identifying urban structures and land use, in the form of LCZ classes, is essential for analyzing and understanding urban heat islands and spatio-temporal dynamics of cities worldwide. We will make use of the freely and publicly available Sentinel-1 and Sentinel-2 datasets of radar and optical images, where one year of global data (4 PB) is available.
The dataset is hand-labelled in a number of classes, e.g., “dense trees”, “heavy industry”, “water”, “open high-rise”, etc. The amount of available data allows for training of deep learning models. In the context of DAPHNE, we aim at streamlining the data preparation, classification, and analysis pipelines at scale, improving the classification accuracy, and developing means of temporal change detection and global data analysis.